
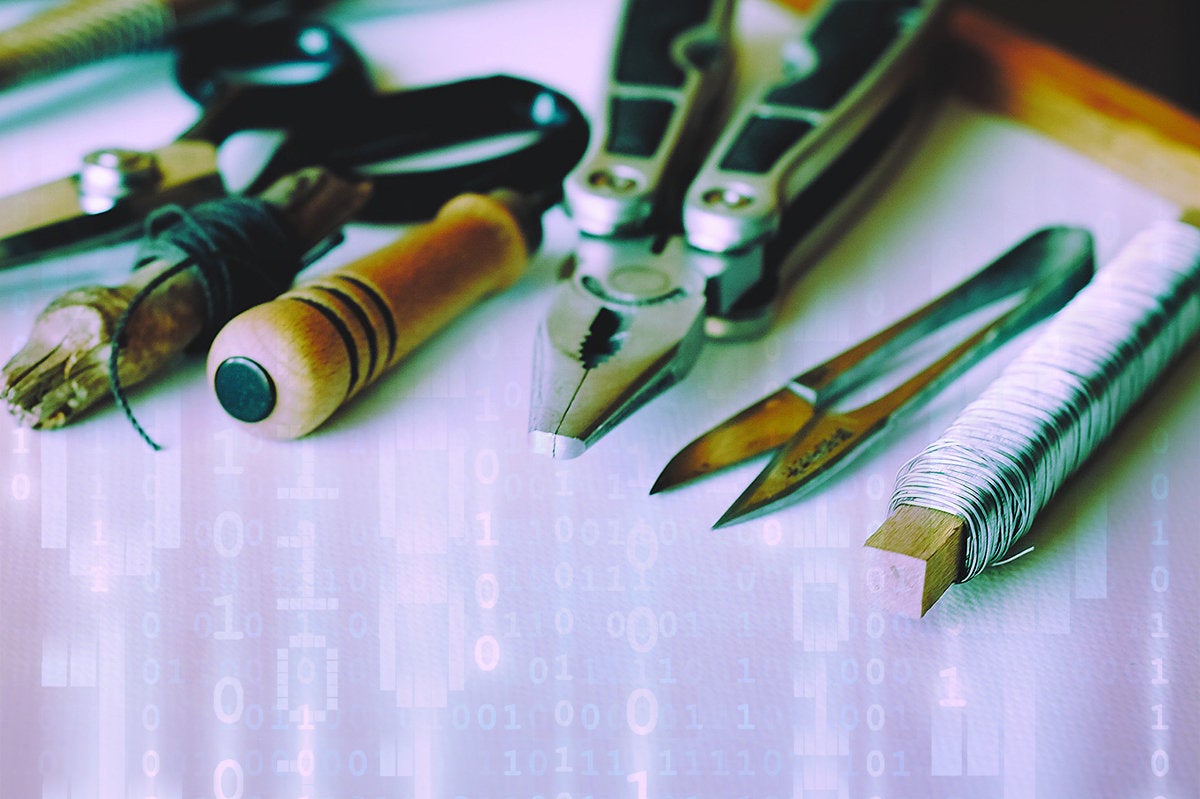
The goal is to explore the data and find some structure within. The system is not told the "right answer." The algorithm must figure out what is being shown. Unsupervised learning is used against data that has no historical labels. For example, it can anticipate when credit card transactions are likely to be fraudulent or which insurance customer is likely to file a claim. Supervised learning is commonly used in applications where historical data predicts likely future events. Through methods like classification, regression, prediction and gradient boosting, supervised learning uses patterns to predict the values of the label on additional unlabeled data. The learning algorithm receives a set of inputs along with the corresponding correct outputs, and the algorithm learns by comparing its actual output with correct outputs to find errors. For example, a piece of equipment could have data points labeled either “F” (failed) or “R” (runs). Supervised learning algorithms are trained using labeled examples, such as an input where the desired output is known. Fraud detection? One of the more obvious, important uses in our world today.Knowing what customers are saying about you on Twitter? Machine learning combined with linguistic rule creation.Online recommendation offers such as those from Amazon and Netflix? Machine learning applications for everyday life.The heavily hyped, self-driving Google car? The essence of machine learning.Here are a few widely publicized examples of machine learning applications you may be familiar with: While many machine learning algorithms have been around for a long time, the ability to automatically apply complex mathematical calculations to big data – over and over, faster and faster – is a recent development. It’s a science that’s not new – but one that has gained fresh momentum. They learn from previous computations to produce reliable, repeatable decisions and results.


It was born from pattern recognition and the theory that computers can learn without being programmed to perform specific tasks researchers interested in artificial intelligence wanted to see if computers could learn from data. The iterative aspect of machine learning is important because as models are exposed to new data, they are able to independently adapt. Because of new computing technologies, machine learning today is not like machine learning of the past.
